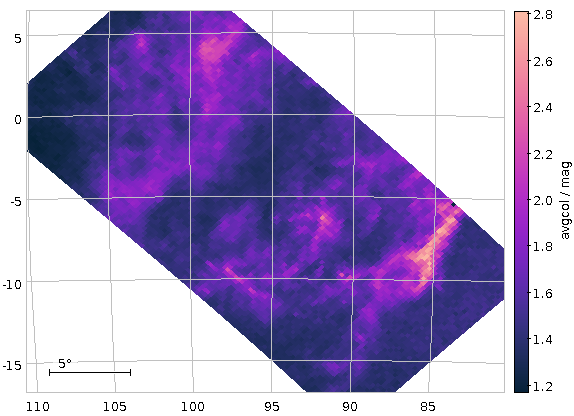
A map of average Gaia colours in HEALPixes 2/83 and 2/86 (Orion south-east). This post tells you how to (relatively) quickly produce such maps.
This year's puzzler for the AG Tagung turned out to be a valuable source of interesting ADQL queries. I have already written about finding dusty spots on the sky, and in the puzzler solution, I had promised some words on creating dust maps, or, more generally, HEALPix maps of any sort.
Making HEALPix maps with Gaia source_ids
The basic technique is explained in Mark Taylor's classical ADASS poster from 2016. On GAVO's TAP service (access URL http://dc.g-vo.org/tap), you will also find an example for that (in TOPCAT's TAP window, check the Service-provided section unter the Examples button for it). However, once you have Gaia source_ids, there is something a lot faster and arguably not much less convenient. Let me quote the footnote on source_id from my DR3 lite table:
For the contents of Gaia DR3, the source ID consists of a 64-bit integer, least significant bit = 1 and most significant bit = 64, comprising:
- a HEALPix index number (sky pixel) in bits 36 - 63; by definition the smallest HEALPix index number is zero.
- […]
This means that the HEALpix index level 12 of a given source is contained in the most significant bits. HEALpix index of 12 and lower levels can thus be retrieved as follows:
- [...]
- HEALpix [at] level n = (source_id)/(235⋅412 − n).
That is: Once you have a Gaia source_id, you an compute HEALpix indexes on levels 12 or less by a simple integer division! I give you that the more-than-35-bit numbers you have to divide by do look a bit scary – but you can always come back here for cutting and pasting:
HEALPix level | Integer-divide source id by |
---|---|
12 | 34359738368 |
11 | 137438953472 |
10 | 549755813888 |
9 | 2199023255552 |
8 | 8796093022208 |
7 | 35184372088832 |
6 | 140737488355328 |
5 | 562949953421312 |
4 | 2251799813685248 |
3 | 9007199254740992 |
2 | 36028797018963968 |
If you know – and that is very valuable knowledge far beyond this particular application – that you can simply jump between HEALPix indexes of different levels by multiplying with or integer-dividing by four, the general formula in the footnote actually becomes rather memorisable. Let me illustrate that with an example in Python. HEALPix number 3145 on level 6 is:
>>> 3145//4 # ...within this HEALPix on level 5... 786 >>> 3145*4, (3145+1)*4 # ..and covers these on level 7... (12580, 12584)
Simple but ingenious.
You can immediately exploit this to make HEALPix maps like the one in the puzzler. This piece of ADQL does the job within a few seconds on the GAVO DC TAP service[1]:
SELECT source_id/8796093022208 AS pix, AVG(phot_bp_mean_mag-phot_rp_mean_mag) AS avgcol FROM gaia.edr3lite WHERE distance(ra, dec, 246.7, -24.5)<2 GROUP by pix
Using the table above, you see that the horrendous 8796093022208 is the code for HEALPix level 8. When you remember (and you should) that HEALPix level 6 corresponds to a linear dimension of about 1 degree and each level is a factor of two in linear dimension, you see that the map ought to have a resolution of about 1/8th of a degree.
HEALPix to Screen Pixel
How do you plot this? Well, in TOPCAT, do Graphics → Sky Plot, and then in the plot window Layers → Add HEALPix control (there are icons for both of these, too). You then have to manually configure the plot for the table you just retrieved: Set the Level to 8, the index to pix and the Value to avgcol – we're working on making the annotation a bit richer so that TOPCAT has a chance to figure this out by itself.
With a bit of extra configuration, you get the following map of average colours (really: dust concentration):
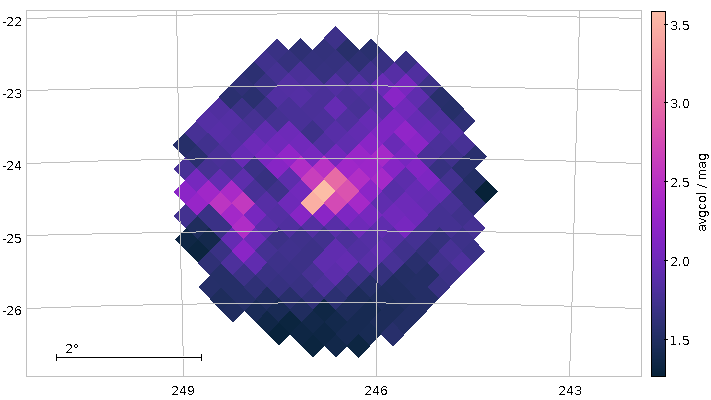
This is not totally ideal, as at the border of the cone, certain Healpixes are only partially covered, which makes statistics unnecessarily harder.
Positional Constraints using source_ids
Due to Gaia's brilliant numbering scheme, we can do analysis by HEALpix, too, circumventing (among other things) this problem. Say you are interested in the vicinity of the M42 and would like to investigate a patch of about 8 degrees. By our rule of thumb, 8 degrees is three levels up from the one-degree level 6. To find the corresponding HEALpix index, on DaCHS servers with their gavo_simbadpoint UDF you could say:
SELECT TOP 1 ivo_healpix_index(3, gavo_simbadpoint('M42')) FROM tap_schema.tables
Hu, you ask, what's tap_schema.tables to do with this? Well, nothing, really. It's just that ADQL's syntax requires selecting from a table, even if what we select is completely independent of any table, as for instance the index of M42's 3-HEALpix. The hack above picks in a table guaranteed to exist on all TAP services, and the TOP 1 makes sure we only compute the value once. In case you ever feel the need to abuse a TAP service as a calculator: Keep this trick in mind.
The result, 334, you could also have found more graphically, as follows:
- Start Aladin
- Check Overlay → HEALPix grid
- Enter M42 in Command
- Zoom out until you see HEALPix indexes of level 3 in the grid.
An advantage you have with this method: You see that M42 happens to lie on a border of HEALPixes; perhaps you should include all of 334, 335, 356, and 357 if you were really interested in the Orion Nebula's vicinity.
We, on the other hand, are just interested in instructive examples, and hence let's just repeat our colour mapping with all Gaia objects from HEALPix 3/334. How do you select these? Well, by source_id's construction, you know their source_ids will be between 334⋅9007199254740992 and (334 + 1)⋅9007199254740992 − 1:
SELECT source_id/8796093022208 AS pix, AVG(phot_bp_mean_mag-phot_rp_mean_mag) AS avgcol FROM gaia.edr3lite WHERE source_id BETWEEN 334*9007199254740992 AND 335*9007199254740992-1 GROUP by pix
This is computationally cheap (though Postgres, not being a column store still has to do quite a bit of I/O; note how much faster this query is when you run it again and all the tuples are already in memory). Even going to HEALPix level 2 would in general still be within our sync time limit. The opening figure was produced with the constraint:
source_id BETWEEN 83*36028797018963968 AND 84*36028797018963968-1 OR source_id BETWEEN 86*36028797018963968 AND 87*36028797018963968-1
– and with a sync query.
Aggregating over a Non-HEALPix
One last point: The constraints we have just been using are, in effect, positional constraints. You can also use them as quick and in some sense rather unbiased sampling tools.
For instance, if you would like so see how the reddening in one of the “dense“ spots in the opening picture behaves with distance, you could first pick a point – α = 98, δ = 4, say –, then convert that to a level 7 healpix as above (that's/88974) and then write:
SELECT ROUND(r_med_photogeo/200)*200 AS distbin, COUNT(*) as n, AVG(phot_bp_mean_mag-phot_rp_mean_mag) AS avgcol FROM gaia.dr3lite JOIN gedr3dist.main USING (source_id) WHERE source_id BETWEEN 88974*35184372088832 and 88975*35184372088832-1 GROUP BY distbin
This is creating 200 pc bins in distance based on the estimates in the gedr3dist.main table (note that this adds subtle correlations, because these estimates already contain Gaia colour information). Since quite a few of these bins will be very sparsely populated, I'm also fetching the number of objects contributing. And then I plot the whole thing, using the conventional √(n) ⁄ n as a rough estimate for the relative error:
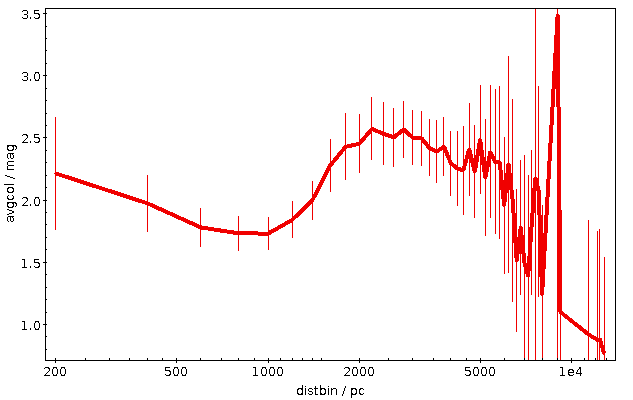
This plot immediatly shows that colour systematics are not exclusively due to dust, as in that case things would only get redder all the time. The blueward trend up to 700 pc is reasonably well explained by the brighter, bluer upper main sequence becoming more dominant in the population sampled as red dwarfs become too faint for Gaia.
The strong reddening setting in after that is rather certainly due to the Orion complex, though I would perhaps not have expected it to reach out to 2 kpc (the conventional distance to M42 is about 0.5 kpc); without having properly thought about it, I'll chalk it off as “the Orion arm“. And after that, it's again what I'd call Malmquist-blueing until the whole things dissolves into noise.
In conclusion: Did you know you can group by both healpix and distbin at the same time? I am sure there are interesting structures to be found in what you will get from such a query…
[1] | You may be tempted to write source_id/(POWER(2, 35)*POWER(4, 3) here for clarity. Resist that temptation. POWER returns floating point numbers. If you have one float in a division, not even a ROUND will get you back into the integer division realm, and the whole trick implodes. No, you will need the integer literals for now. |