Global Dataset Discovery in PyVO
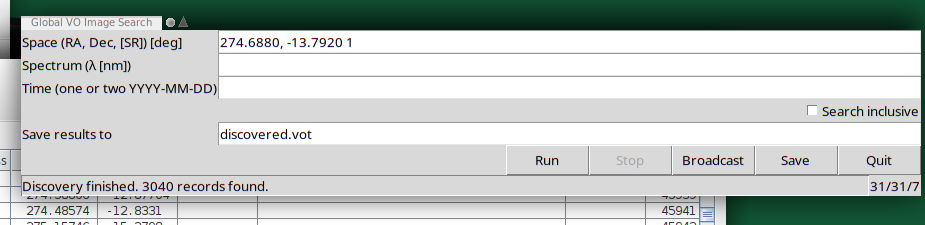
Admittedly somewhat old-style: As part of teaching global dataset discovery to pyVO, I have also come up with a Tkinter GUI for it. See A UI for more on this.
One of the more exciting promises of the Virtual Observatory was global dataset discovery: You say “Give me all spectra of object X that there are“, and the computer relates that request to all the services that might have applicable data. Once the results come in, they are merged into some uniformly browsable form.
In the early VO, there were a few applications that let you do this; I fondly remember VODesktop. As the VO grew and diversified, however, this became harder and harder, partly because there were more and more services, partly because there were more protocols through which to publish data. Thus, for all I can see, there is, at this point, no software that can actually query all services plausibly serving, say, images or spectra in the VO.
I have to say that writing such a thing is not for the faint-hearted, either. I probably wouldn't have tackled it myself unless the pyVO maintainers had made it an effective precondition for cleaning up the pyVO Servicetype constraint.
But they did, and hence as a model I finally wrote some code to do all-VO image searches using all of SIA1, SIA2, and obscore, i.e., the two major versions of the Simple Image Access Protocol plus Obscore tables published through TAP services. I actually have already reported in Tucson on some preparatory work I did last summer and named a few problems:
- There are too many services to query on a regular basis, but filtering them would require them to declare their coverage; far too many still don't.
- With the current way of registering obscore tables, there is no way to know their coverage.
- One dataset may be availble through up to three protocols on a single host.
- SIA1 does not even let you constrain time and spectrum.
Some of these problems I can work around, others I can try to fix. Read on to find out how I fared so far.
The pyVO API
Currently, the development happens in pyVO PR #470. While it is still a PR, let me point you to temporary pyVO docs on the proposed pyvo.discover module – of course, all of this is for review and probably not in the shape it will remain in[1].
To quote from there, the basic usage would be something like:
from pyvo import discover from astropy import units as u from astropy import time datasets, log = discover.images_globally( space=(274.6880, -13.7920, 0.1), spectrum=500.7*u.nm, time=(time.Time('1995-01-01'), time.Time('1995-12-31')))
At this point, only a cone is supported as a space constraint, and only a single point in spectrum. It would certainly be desirable to be more flexible with the space constraint, but given the capabilities of the various protocols, that is hard to do. Actually, even with the plain cone Obscore (i.e., ironically, the most powerful of the discovery protocols covered here) currently results in an implementation that makes me unhappy: ugly, slow, and wrong. This is requires a longer discussion; see Appendix: Optionality Considered Harmful.
datasets at this point is a list of, conceputally, Obscore records. Technically, the list contains instances of a custom class ImageFound, which have attributes named after the Obscore columns. In case you have doubts about the Semantics of any column, the Obscore specification is there to help. And yes, you can argue we should create a single astropy table from that list. You are probably right.
PyVO adds an extra column over the mandatory obscore set, origin_service. This contains the IVOA identifier (IVOID) of the service at which the dataset was found. You have probably seen IVOIDs before: they are URIs with a scheme of ivo:. What you may not know: these things actually resolve, specifically to registry resource records. You can do this resolution in a web browser: Just prepend https://dc.g-vo.org/I/ to an IVOID and paste the result into the address bar. For instance, my Obscore table has the IVOID ivo://org.gavo.dc/__system__/obscore/obscore; the link below the IVOID leads you to an information page, which happens to be the resource's Registry record formatted with a bit of XSLT. A somewhat more readable but less informative rendering is available when you prepend https://dc.g-vo.org/LP/ (“landing page”).
The second value returned from discover.images_globally is a list of strings with information on how the global discovery progressed. For now, this is not intended to be machine-readable. Humans can figure out which resources were skipped because other services already cover their data, which services yielded how many records, and which services failed, for instance:
Skipping ivo://org.gavo.dc/lswscans/res/positions/siap because it is served by ivo://org.gavo.dc/__system__/obscore/obscore Skipping ivo://org.gavo.dc/rosat/q/im because it is served by ivo://org.gavo.dc/__system__/obscore/obscore Obscore GAVO Data Center Obscore Table: 2 records SIA2 The VO @ ASTRON SIAP Version 2 Service: 0 records SIA2 ivo://au.csiro/casda/sia2 skipped: ReadTimeout: HTTPSConnectionPool(host='casda.csiro.au', port=443): Read timed out. (read timeout=20) SIA2 CADC Image Search (SIA): 0 records SIA2 European HST Archive SIAP service: 0 records ...
(On the skipping, see Relationships below). I consider this crucial provenance, as that lets you assess later what you may have missed. When you save the results, be sure to save these, too.
A feature that will presumably (see Inclusivity for the reasons for this expectation) be important at least for a few years is that you can pass the result of a Registry query, and pyVO will try to find services suitable for image discovery on that set of resources.
A relatively straightforward use case for that is global obscore discovery. This would look like this:
from pyvo import discover from pyvo import registry from astropy import units as u from astropy import time def say(s): print(s) datasets, log = discover.images_globally( space=(274.6880, -13.7920, 1), time=(time.Time('1995-01-01'), time.Time('1995-12-31')), services=registry.search(registry.Datamodel("obscore")), watcher=say)
(the watcher thing lets you, well, watch the progress of the discovery).
A UI
To get an idea whether this API might one day work for the average astronomer, I have written a Tkinter-based GUI to global image discovery as it is now: tkdiscover (only available from github at this point). This is what a session with it might look like:
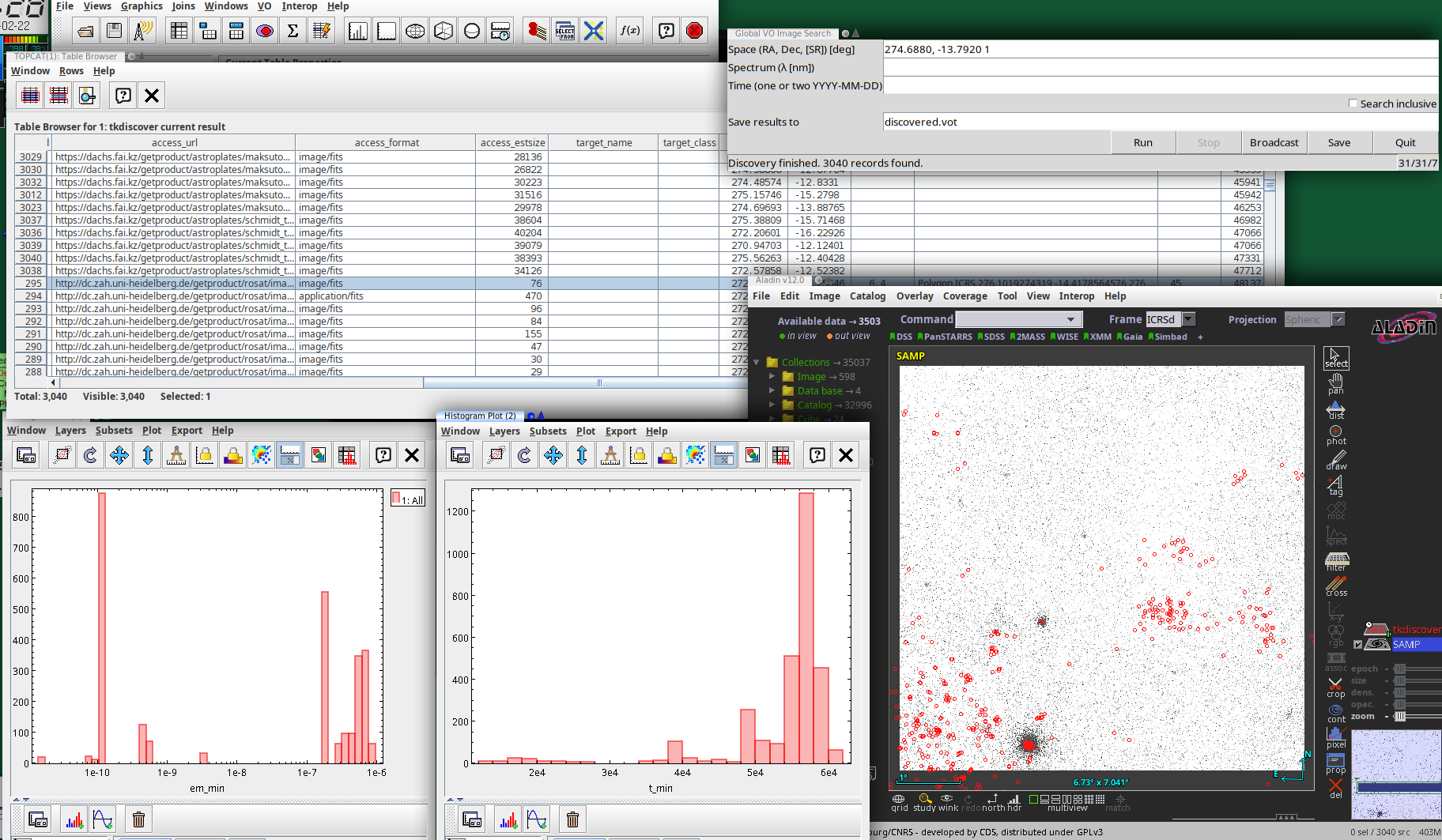
The actual UI is in the top right: A plain window in which you can configure a global discovery query by straightfoward serialisations of discover.images_globally's arguments:
- Space (currently, a cone in RA, Dec, and search radius, separated by whitespace of commas)
- Spectrum (currently, a single point as a wavelength in metres)
- Time (currently, either a single point in time – which probably is rarely useful – or an interval, to be entered as civil DALI dates
- Inclusivity.
When you run this, this basically calls discover.images_globally and lets you know how it is progressing. You can click Broadcast (which sends the current result to all VOTable clients on the SAMP bus) or Save at any time and inspect how discovery is progressing. I predict you will want to do that, because querying dozens of services will take time.
There is also a Stop button that aborts the dataset search (you will still have the records already found). Note that the Stop button will not interrupt running network operations, because the network library underneath pyVO, requests, is not designed for being interrupted. Hence, be patient when you hit stop; this may take as long as the configured timeout (currently is 20 seconds) if the service hangs or has to do a lot of work. You can see that tkdiscover has noticed your stop request because the service counter will show a leading zero.
Service counter? Oh, that's what is at the bottom right of the window. Once service discovery is done, that contains three numbers: The number of services to query, the number of services queried already, and the number of services that failed.
The table contains the obscore records described above, and the log lines are in the discovery_log INFO. I will give you that this is extremely unreadable in particular in TOPCAT, which normalises the line separators to plain whitespace. Perhaps some other representation of these log lines would be preferable: A PARAM with a char[][] (but VOTable still is terrible with arrays of variable-length strings)? Or a separate table with char[*] entries?
Inclusivity
I have promised above I'd explain the “Inclusive” part in both the pyVO API and the Tk UI. Well, this is a bit of a sad story.
All-VO-queries take time. Thus, in pyVO we try to only query services that we expect serve data of interest. How do we arrive at expectations like that? Well, quite a few records in the Registry by now declare their coverage in space and time (cf. my 2018 post for details).
The trouble is: Most still don't. The checkmark at inclusive decides whether or not to query these “undecidable” services. Which makes a huge difference in runtime and effort. With the pre-configured constraints in the current prototype (X-Ray images a degree around 274.6880, -13.7920 from the year 1995), we currently discover three services (of which only one actually needs to be queried) when inclusive is off. When it is on, pyVO will query a whopping 323 services (today).
The inclusivity crisis is particularly bad with Obscore tables because of their broken registration pattern; I can say that so bluntly because I am the author of the standard at fault, TAPRegExt. I am preparing a note with a longer explanation and proposals for fixing matters – <cough> follow me on github –, but in all brevity: Obscore data is discovered using something like a flag on TAP services. That is bad because the TAP services usually have entriely different metadata from their Obscore table; think, in particular, of the physical coverage that is relevant here.
It will be quite a bit of effort to get the data providers to do the Registry work required to improve this situation. Until that is done, you will miss Obscore tables when you don't check inclusive (or override automatic resource selection as above) – and if you do check inclusive, your discovery runs will take something like a quarter of an hour.
Relationships
In general, the sheer number of services to query is the Achilles' heel in the whole plan. There is nothing wrong with having a machine query 20 services, but querying 200 is starting to become an effort.
With multi-data collection services like Obscore (or collective SIA2 services), getting down to a few dozen services globally for a well-constrained search is actually not unrealistic; once all resources properly declare their coverage, it is not very likely that more than 20 institutions worldwide will have data in a credibly small region of space, time, and spectrum. If all these run collective services and properly declare the datasets to be served by them, that's our 20-services global query right there.
However, pyVO has to know when data contained in a resource is actually queriable by a collective service. Fortunately, this problem has already been addressed in the 2019 endorsed note on Discovering Data Collections Within Services: Basically, the individual resource declares an IsServedBy relationship to the collective service. PyVO global discovery already looks at these. That is how it could figure out these two things in the sample log given above:
Skipping ivo://org.gavo.dc/lswscans/res/positions/siap because it is served by ivo://org.gavo.dc/__system__/obscore/obscore Skipping ivo://org.gavo.dc/rosat/q/im because it is served by ivo://org.gavo.dc/__system__/obscore/obscore
But of course the individual services have to declare these relationships. Surprisingly many already do, as you can observe yourself when you run:
select ivoid, related_id from rr.relationship natural join rr.capability where standard_id like 'ivo://ivoa.net/std/sia%' and relationship_type='isservedby'
on your favourite RegTAP endpoint (if you have no preferences, use mine: http://dc.g-vo.org/tap). If you have collective services and run individual SIA services, too, please run that query, see if you are in there, and if not, please declare the necessary relationships. In case you are unsure as to what to do, feel free to contact me.
Future Directions
At this point, this is a rather rough prototype that needs a lot of fleshing out. I am posting this in part to invite the more adventurous to try (and break) global discovery and develop further ideas.
Some extensions I am already envisaging include:
Write a similar module for spectra based on SSAP and Obscore. That would then probably also work for time series and similar 1D data.
Do all the Registry work I was just talking about.
Allow interval-valued spectral constraints. That's pretty straightforward; if you are looking for some place to contribute code, this is what I'd point you to.
Track overflow conditions. That should also be simple, probably just a matter of perusing the pyVO docs or source code and then conditionally produce a log entry.
Make an obscore s_region out of the SIA1 WCS information. This should also be easy – perhaps someone already has code for that that's tested around the poles and across the stitching line? Contributions are welcome.
Allow more complex geometries to define the spatial region of interest. To keep SIA1 viable in that scenario it would be conceivable to compute a bounding box for SIA1 POS/SIZE and do “exact” matching locally on the coarser SIA1 result.
Enable multi-position or multi-interval constraints. This pretty certainly would exclude SIA1, and, realistically, I'd probably only enable Obscore services with TAP uploads with this. With those constraints, it would be rather straightforward.
Add SODA support: It would be cool if my ImageFound had a way to say “retrieve data for my RoI only”. This would use SODA and datalink to do server-side cutouts where available and do the cut-out locally otherwise. If this sounds like rocket science: No, the standards for that are actually in place, and pyVO also has the necessary support code. But still the plumbing is somewhat tricky, partly also because pyVO's datalink API still is a bit clunky.
Going async? Right now, we civilly query one service after the other, waiting for each result before proceeding to the next service. This is rather in line with how pyVO is written so far.
However, on the network side for many years asynchronous programming has been a very successful paradigm – for instance, our DaCHS package has been based on an async framework from the start, and Python itself has growing in-language support for async, too.
Async allows you to you fire off a network request and forget about it until the results come back (yes, it's the principle of async TAP, too). That would let people run many queries in parallel, which in turn would result in dramatically reduced waiting times, while we can rather easily ensure that a single client will not overflow any server. Still, it would be handing a fairly powerful tool into possibly unexperienced hands… Well: for now there is no need to decide on this, as pyVO would need rather substantial upgrades to support async.
Appendix: Optionality Considered Harmful
The trouble with obscore and cones is a good illustration of the traps of attempting to fix problems by adding optional features. I currently translate the cone constraint on Obscore using:
"(distance(s_ra, s_dec, {}, {}) < {}".format( self.center[0], self.center[1], self.radius) +" or 1=intersects(circle({}, {}, {}), s_region))".format( self.center[0], self.center[1], self.radius))
which is all of ugly, presumably slow, and wrong.
To appreciate what is going on, you need to know that Obscore has two ways to define the spatial coverage of an observation. You can give its “center” (s_ra, s_dec) and something like a rough radius (s_fov), or you can give some sort of geometry (e.g., a polygon: s_region). When the standard was written, the authors wanted to enable Obscore services even on databases that do not know about spherical geometry, and hence s_region is considered rather optional. In consequence, it is missing in many services. And even the s_ra, s_dec, s_fov combo is not mandatory non-null, so you are perfectly entitled to only give s_region.
That is why there are the two conditions or-ed together (ugly) in the code fragment above. 1=intersects(circle(.), s_region) is the correct part; this is basically how the cone is interpreted in SIA1, too. But because s_region may be NULL even when s_ra and s_dec are given, we also need to do a test based on the center position and the field of view. That rather likely makes things slower, possibly quite a bit.
Even worse, the distance-based condition actually is wrong. What I really ought to take into account is s_fov and then do something like distance(.) < {self.radius}+s_fov, that is, the dataset position need only be closer than the cone radius plus the dataset's FoV (“intersects”). But that would again produce a lot of false negatives because s_fov may be NULL, too, and often is, after which the whole condition would be false.
On top of that, it is virtually impossible that such an expression would be evaluated using an index, and hence with this code in place, we would likely be seqscanning the entire obscore table almost every time – which really hurts when you have about 85 Million records in your Obscore table (as I do).
The standard could immediately have sanitised all this by saying: when you have s_ra and s_dec, you must also give a non-empty s_fov and s_region. This is a classic case for where a MUST would have been necessary to produce something that is usable without jumping through hoops. See my post on Requirements and Validators on this blog for a longer exposition on this whole matter.
I'm not sure if there is a better solution than the current “if the operators didn't bother with s_region, the dataset's FoV will be ignored“. If you have good ideas, by all means let me know.
[1] | If you want to try this (in particular without clobbering your “normal” pyVO), do something like this: virtualenv --system-site-packages global-datasets . global-datasets/bin/activate cd global-datasets git clone https://github.com/msdemlei/pyvo cd pyvo git checkout global-datasets pip install . |