A New Constraint Class in PyVO's Registry API: UAT
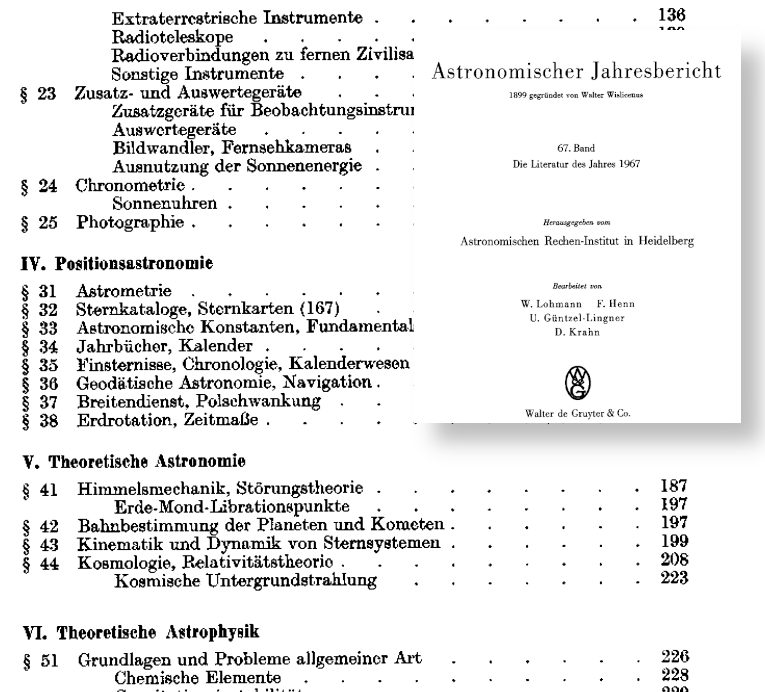
This was how they did what I am talking about here almost 60 years ago: a page of the table of contents of the “Astronomischer Jahresbericht” for 1967, the last volume before it was turned into the English-language Astronomy and Astrophysics Abstracts, which were the main tool for literature work in astronomy until the ADS came along in the late 1990ies.
I have recently created a pull request against pyVO to furnish the library with a new constraint to search for data and services: Search by a concept drawn from the Unified Astronomy Thesaurus UAT. This is not entirely different from the classical search by subject keywords that was what everyone did before we had the ADS, which is what I am trying to illustrate above. But it has some twists that, I would argue, still make it valuable even in the age of full-text indexes.
To make my argument, let me first set the stage.
Thesauri and the UAT
(Disclaimer: I am currently a member of the UAT steering committee and therefore cannot claim neutrality. However, I would not claim neutrality otherwise, either: the UAT is not perfect, but it's already great)
Librarians (and I am one at heart) love thesauri. Or taxonomies. Or perhaps even ontologies. What may sound like things out of a Harry Potter novel are actually ways to organise a part of the world (a “domain”) into “concepts”. If you are suitably minded, you can think of a “concept“ as a subset of the domain; “suitably minded“ here means that you consider the world as a large set of things and a domain a subset of this world. The IVOA Vocabularies specification contains some additional philosophical background on this way of thinking in sect. 5.2.4.
On this other hand, if you are not suitably minded, a “concept” is not much different from a topic.
There are differences in how each of thesaurus, taxonomy, and ontology does that organising (and people don't always agree on the differences). Ontologies, for instance, let you link concepts in every way, as in “a (bicycle) (is steered) (using) a (handle bar) (made of) ((steel) or (aluminum))“; every parenthesised phrase would be a node (which is a better term in ontologies than “concept”) in a suitably general ontology, and connecting these nodes creates a fine-graned representation of knowledge about the world.
That is potentially extremely powerful, but also almost too hard for humans. Check out WordNet for how far one can take ontologies if very many very smart people spend very many years.
Thesauri, on the other hand, are not as powerful, but they are simpler and within reach for mere humans: there, concepts are simply organised into something like a tree, perhaps (and that is what many people would call a taxonomy) using is-a relationships: A human is a primate is a mammal is a vertebrate is an animal. The UAT actually is using somewhat vaguer notions called “narrower” and “wider”. This lets you state useful if somewhat loose relationships like “asteroid-rotation is narrower than asteroid-dynamics”. For experts: The UAT is using a formalism called SKOS; but don't worry if you can't seem to care.
The UAT is standing on the shoulders of giants: Before it, there has been the IAU thesaurus in 1993, and an astronomy thesaurus was also produced under the auspices of the IVOA. And then there were (and to some extent still are) the numerous keyword schemes designed by journal publishers that would also count as some sort of taxonomy or astronomy.
“Numerous” is not good when people have to assign keywords to their journal articles: If A&A use something drastically or only subtly different from ApJ, and MNRAS still something else, people submitting to multiple journals will quite likely lose their patience and diligence with the keywords. For reasons I will discuss in a second, that is a shame.
Therefore, at least the big American journals have now all switched to using UAT keywords, and I sincerely hope that their international counterparts will follow their example where that has not already happened.
Why Keywords?
Of course, you can argue that when you can do full-text searches, why would you even bother with controlled keyword lists? Against that, I would first argue that it is extremely useful to have a clear idea of what a thing is called: For example, is it delta Cephei stars, Cepheids, δ Cep stars or still something else? Full text search would need to be rather smart to be able to sort out terminological turmoil of this kind for you.
And then you would still not know if W Virginis stars (or should you say “Type II Cepheids”? You see how useful proper terminology is) are included in whatever your author called Cepheids (or whatever they called it). Defining concepts as precisely as possible thus is already great.
The keyword system becomes even more useful when the hiearchy we see in the Cepheid example becomes visible to computers. If a computer knows that there is some relationship between W Virgins stars and classical Cepheids, it can, for instance, expand or refine your queries (“give me data for all kinds of Cepheids”) as necessary. To give you an idea of how this looks in practice, here is how SemBaReBro displays the Cepheid area in the UAT:
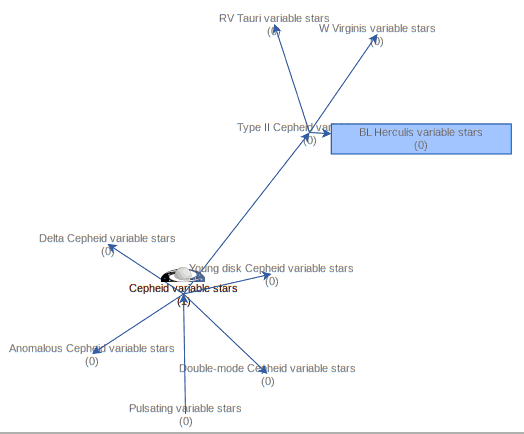
In that image, only concepts associated with resources in the Registry have a spiffy IVOA logo; that so few VO resources claim to deal with Cepheids tells you that our data providers can probably improve their annotations quite a bit. But that is for another day; the hope is that as more people search using UAT concepts, the data providers will see a larger benefit in choosing them wisely[1].
By the way, if you are a regular around here, you will have seen images like that before; I have talked about Sembarebro in 2021 already, and that post contains more reasons for having and maintaining vocabularies.
Oh, and for the definitions of the concepts, you can (in general; in the UAT, there are still a few concepts without definitions) dereference the concept URI, which in the VO is always of the form <vocabulary uri>#<term identifier>, where the vocabulary URI starts with http://www.ivoa.net/rdf, after which there is the vocabulary name.
Thus, if you point your web browser to https://www.ivoa.net/rdf/uat#cepheid-variable-stars[2], you will learn that a Cepheid is:
A class of luminous, yellow supergiants that are pulsating variables and whose period of variation is a function of their luminosity. These stars expand and contract at extremely regular periods, in the range 1-50 days [...]
The UAT constraint
Remember? This was supposed to be a blog post about a new search constraint in pyVO. Well, after all the preliminaries I can finally reveal that once pyVO PR #649 is merged, you can search by UAT concepts:
>>> from pyvo import registry >>> print(registry.search(registry.UAT("variable-stars"))) <DALResultsTable length=2010> ivoid ... ... object ... --------------------------------- ... ivo://cds.vizier/b/corot ... ivo://cds.vizier/b/gcvs ... ivo://cds.vizier/b/vsx ... ivo://cds.vizier/i/280b ... ivo://cds.vizier/i/345 ... ivo://cds.vizier/i/350 ... ... ... ivo://cds.vizier/v/97 ... ivo://cds.vizier/vii/293 ... ivo://org.gavo.dc/apass/q/cone ... ivo://org.gavo.dc/bgds/l/meanphot ... ivo://org.gavo.dc/bgds/l/ssa ... ivo://org.gavo.dc/bgds/q/sia ...
In case you have never used pyVO's Registry API before, you may want to skim my post on that topic before continuing.
Since the default keyword search also queries RegTAP's res_subject table (which is what this constraint is based on), this is perhaps not too exciting. At least there is a built-in protection against typos:
>>> print(registry.search(registry.UAT("varialbe-stars"))) Traceback (most recent call last): File "<stdin>", line 1, in <module> File "/home/msdemlei/gavo/src/pyvo/pyvo/registry/rtcons.py", line 713, in __init__ raise dalq.DALQueryError( pyvo.dal.exceptions.DALQueryError: varialbe-stars does not identify an IVOA uat concept (see http://www.ivoa.net/rdf/uat).
It becomes more exciting when you start exploiting the intrinsic hierarchy; the constraint constructor supports optional keyword arguments expand_up and expand_down, giving the number of levels of parent and child concepts to include. For instance, to discover resources talking about any sort of supernova, you would say:
>>> print(registry.search(registry.UAT("supernovae", expand_down=10))) <DALResultsTable length=593> ivoid ... ... object ... ---------------------------------------- ... ivo://cds.vizier/b/sn ... ivo://cds.vizier/ii/159 ... ivo://cds.vizier/ii/189 ... ivo://cds.vizier/ii/205 ... ivo://cds.vizier/ii/214a ... ivo://cds.vizier/ii/218 ... ... ... ivo://cds.vizier/j/pasp/122/1 ... ivo://cds.vizier/j/pasp/131/a4002 ... ivo://cds.vizier/j/pazh/30/37 ... ivo://cds.vizier/j/pazh/37/837 ... ivo://edu.gavo.org/eurovo/aida_snconfirm ... ivo://mast.stsci/candels ...
There is no overwhelming magic in this, as you can see when you tell pyVO to show you the query it actually runs:
>>> print(registry.get_RegTAP_query(registry.UAT("supernovae", expand_down=10))) SELECT [crazy stuff elided] WHERE (ivoid IN (SELECT DISTINCT ivoid FROM rr.res_subject WHERE res_subject in ( 'core-collapse-supernovae', 'hypernovae', 'supernovae', 'type-ia-supernovae', 'type-ib-supernovae', 'type-ic-supernovae', 'type-ii-supernovae'))) GROUP BY [whatever]
Incidentally, some services have an ADQL extension (a “user defined function“ or UDF) that lets you do these kinds of things on the server side; that is particularly nice when you do not have the power of Python at your fingertips, as for instance interactively in TOPCAT. This UDF is:
gavo_vocmatch(vocname STRING, term STRING, matchagainst STRING) -> INTEGER
(documentation at the GAVO data centre). There are technical differences, some of which I try to explain in amoment. But if you run something like:
SELECT ivoid FROM rr.res_subject WHERE 1=gavo_vocmatch('uat', 'supernovae', res_subject)
on the TAP service at http://dc.g-vo.org/tap, you will get what you would get with registry.UAT("supernovae", expand_down=1). That UDF also works with other vocabularies. I particularly like the combination of product-type, obscore, and gavo_vocmatch.
If you wonder why gavo_vocmatch does not go on expanding towards narrower concepts as far as it can go: That is because what pyVO does is semantically somewhat questionable.
You see, SKOS' notions of what is wider and narrower are not transitive. This means that just because A is wider than B and B is wider than C it is not certain that A is wider than C. In the UAT, this sometimes leads to odd results when you follow a branch of concepts toward narrower concepts, mostly because narrower sometimes means part-of (“Meronymy”) and sometimes is-a (“Hyponymy“). Here is an example discovered by my colleague Adrian Lucy:
interstellar-medium wider nebulae wider emission-nebulae wider planetary-nebulae wider planetary-nebulae-nuclei
Certainly, nobody would argue that that the central stars of planetary nebulae somehow are a sort of or are part of the interstellar medium, although each individual relationship in that chain makes sense as such.
Since SKOS relationships are not transitive, gavo_vocmatch, being a general tool, has to stop at one level of expansion. By the way, it will not do that for the other flavours of IVOA vocabularies, which have other (transitive) notions of narrower-ness. With the UAT constraint, I have fewer scruples, in particular since the expansion depth is under user control.
Implementation
Talking about technicalities, let me use this opportunity to invite you to contribute your own Registry constraints to pyVO. They are not particularly hard to write if you know both ADQL and Python. You will find several examples – between trivial and service-sensing complex in pyvo.registry.rtcons. The code for UAT looks like this (documentation removed for clarity[3]):
class UAT(SubqueriedConstraint): _keyword = "uat" _subquery_table = "rr.res_subject" _condition = "res_subject in {query_terms}" _uat = None @classmethod def _expand(cls, term, level, direction): result = {term} new_concepts = cls._uat[term][direction] if level: for concept in new_concepts: result |= cls._expand(concept, level-1, direction) return result def __init__(self, uat_keyword, *, expand_up=0, expand_down=0): if self.__class__._uat is None: self.__class__._uat = vocabularies.get_vocabulary("uat")["terms"] if uat_keyword not in self._uat: raise dalq.DALQueryError( f"{uat_keyword} does not identify an IVOA uat" " concept (see http://www.ivoa.net/rdf/uat).") query_terms = {uat_keyword} if expand_up: query_terms |= self._expand(uat_keyword, expand_up, "wider") if expand_down: query_terms |= self._expand(uat_keyword, expand_down, "narrower") self._fillers = {"query_terms": query_terms}
Let me briefly describe what is going on here. First, we inherit from the base class SubqueriedConstraint. This is a class that takes care that your constraints are nicely encapsulated in a subquery, which generally is what you want in pyVO. Calmly adding natural joins as recommended by the RegTAP specification is a dangerous thing for pyVO because as soon as a resource matches your constraint more than once (think “columns with a given UCD”), the RegistryResult lists in pyVO will turn funny.
To make a concrete SubqueriedConstraint, you have to fill out:
- the table it will operate on, which is in the _subquery_table class attribute;
- an expression suitable for a WHERE clause in the _condition attribute, which is a template for str.format. This is often computed in the constructor, but here it is just a constant expression and thus works fine as a class attribute;
- a mapping _fillers mapping the substitutions in the _condition string template to Python values. PyVO's RegTAP machinery will worry about making SQL literals out of these, so feel free to just dump Python values in there. See the make_SQL_literal for what kinds of types it understands and expand it as necessary.
There is an extra class attribute called _keyword. This is used by the pyvo.regtap machinery to let users say, for instance, registry.search(uat="foo.bar") instead of registry.search(registry.UAT("foo.bar")). This is a fairly popular shortcut when your constraints can be expressed as simple strings, but in the case of the UAT constraint you would be missing out on all the interesting functionality (viz., the query expansion that is only available through optional arguments to its constructor).
This particular class has some extra logic. For one, we cache a copy of the UAT terms on first use at the class level. That is not critical for performance because caching already happens at the level of get_vocabulary; but it is convenient when we want query expansion in a class method, which in turn to me feels right because the expansion does not depend on the instance. If you don't grok the __class__ magic, don't worry. It's a nerd thing.
More interesting is what happens in the _expand class method. This takes the term to expand, the number of levels to go, and whether to go up or down in the concept trees (which are of the computer science sort, i.e., with the root at the top) in the direction argument, which can be wider or narrower, following the names of properties in Desise, the format we get our vocabulary in. To learn more about Desise, see section 3.2 of Vocabularies in the VO 2.
At each level, the method now collects the wider or narrower terms, and if there are still levels to include, calls itself on each new term, just with level reduced by one. I consider this a particularly natural application of recursion. Finally. everything coming back is merged into a set, which then is the return value.
And that's really it. Come on: write your own RegTAP constraints, and also have fun with vocabularies. As you see here, it's really not that magic.
[1] | Also, just so you don't leave with the impression I don't believe in AI tech at all, something like SciX's KAILAS might also help improving Registry subject keywords. |
[2] | Yes, in a little sleight of hand, I've switched the URI scheme to https here. That's not really right, because the term URIs are supposed to be opaque, but some browsers currently forget the fragment identifiers when the IVOA web server redirects them to https, and so https is safer for this demonstration. This is a good example of why the web would be a better place if http had been evolved to support transparent, client-controlled encryption (rather than inventing https). |
[3] | I've always wanted to write this. |