Semantics, Cross-Discipline Discovery, and Down-To-Earth Code
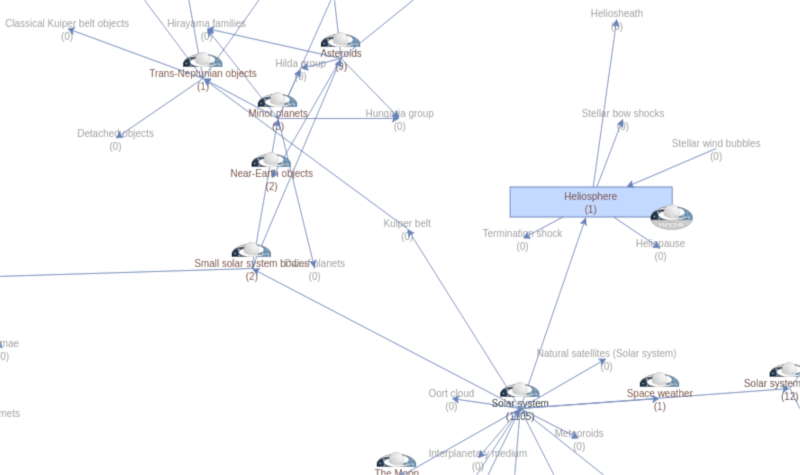
A tiny piece of the Unified Astronomy Thesaurus as viewed by Sembarebro – the IVOA logos sit on terms that have VO resoures on them.
Sometimes people ask me (in particular when I'm wearing my hat as the current chair of the IVOA Semantics working group) “well, what's this semantics thing good for?“ There are many answers, but here's one that nicely meshes with my pet subject data discovery: You want hierarchical, agreed-upon word lists to bridge discipline gaps.
This story starts with B2FIND, a cross-disciplinary metadata aggregator for science data run within the framework of the European Open Science Cloud (EOSC). GAVO (or, more precisely, Heidelberg University's Astronomy) is involved in the EOSC via the ESCAPE project, and so I have had the pleasure of interacting with B2FIND for a while now. In particular, they are harvesting the metadata records of the Virtual Observatory Registry from us.
This of course requires a bit of mapping, because the VO's metadata formats (VOResource, VODataService, and several extensions; see 2014A&C.....7..101D to learn more) are far too fine-grained for the wider scientific public. Not even our good friends from high-energy physics would appreciate being served links to, say, TAP endpoints (yet!). So, on our end we're mapping to the Datacite metadata kernel, which from VOResource is just a piece of XSL away (plus some perhaps debatable conventions).
But there's more to this mapping, such as vocabularies of subject keywords. You might argue that in the age of rapid full text searches, keywords are dead. I would beg to disagree. For example, with good, hierarchical keyword systems you can, among many other useful things, offer topical browsing of metadata repositories. While it might not quite qualify as “useful” yet, the SemBaReBro registry browser I've hacked together late last year would be an example for such facilities – and might become part of our WIRR Registry searching tool one day.
On the topic of subject keywords VOResource says that resources in the VO should be using the Unified Astronomy Thesaurus, specifically in its IVOA incarnation (not quite true yet, but true enough by blog standards). While few do, I've done a mapping of existing keywords in the VO to UAT concepts, which is what's behind SemBaReBro. So: most VO resources now have UAT concepts.
However, these include concepts like AM Canum Venaticorum Stars, which outside of rather specialised circles of astronomers few people will ever have heard about (which, don't get me wrong, I personally regret – they're funky star systems). Hence, B2FIND does not bother with those.
When we discussed the subject mapping for B2FIND, we thought using the UAT's top-level concepts might be a good start. However, at that point no VO resources at all actually used these, and, indeed, within astronomy that generally wouldn't make a lot of sense, because they are to unspecific to help much within the discipline. I postponed and then forgot about the problem – when the keywords of the resources weren't even from UAT, solving the granularity mismatch just wasn't humanly possible.
That was the state of affairs until last Tuesday, when I had a mumble session with B2FIND folks and the topic came up again. And now, thanks partly to the new desise format proposed in the current Vocabularies in the VO 2 draft, things fell nicely into place: Hey, I have UAT concepts, and mapping these to the top-level terms isn't hard either any more.
So, B2FIND gets the toplevel keywords they've been expecting all the time starting today. Yes: This isn't a panacea suddenly solving all the problems of cross-discipline data discovery, not the least because it's harder than one might think to imagine how such a thing would look like in practice. But given the complexities involved I was positively surprised how easy this particular part of the equation was.
From here on, there's a bit of tech babble I intend to re-use in the RFC of Vocabularies in the VO 2; don't feel bad if you skip it.
The first step was to make the mapping from UAT terms to the toplevel terms. The interesting part of the source I'm linking to here is:
def get_roots_for(term, uat_terms): roots, seen = set(), set() def follow(t): wider = uat_terms[t]["wider"] if not wider: if not t in ROOT_TERMS: raise Exception( f"{t} found as a top-level term") roots.add(t) else: seen.add(t) for wider in uat_terms[t]["wider"]: follow(wider) follow(term) return roots
There, uat_terms is essentially just a json-decode of what you get from the vocabulary URI if you ask for desise (see the draft spec linked to above for the technicalities). That's really it, and it even defends against cycles in the concept graph (which are legal by SKOS but shouldn't happen in the UAT) and detached terms (i.e., ones that are not rooted in the top-level terms). For what it does, I claim that's remarkably compact code.
Once I had that, I needed to get the UAT-mapped subject keywords for the records I'm serving to datacite and fiddle the corresponding roots back in. That's technically a bit more involved because I am producing the datacite records on the fly from the XML representation for VOResource records that I keep in the database, and there's a bit of namespace magic involved (full code). Plus, the UAT-mapped keywords are only kept in the database, not in the metadata records.
Still, the core operation here is relatively straightforward. Consider:
def addUATToplevels(dataciteTree): # dataciteTree is an (lxml) ElementTree for the # result of the XSL transformation. That's all # I have, and thus I first have to fiddle out # the identifier we are talking about ivoid = dataciteTree.xpath( "//d:alternateIdentifier[" "@alternateIdentifierType='ivoid']", namespaces={"d": DATACITE_NS} )[0].text.lower() # The .lower() is necessary because ivoids # unfortunately are case-insensitive, and RegTAP # normalises them to lowercase to retain sanity. # Now pull the UAT-mapped subject keywords from # our RegTAP extension (getTableConn is # DaCHS-internal API, but there's no magic in # there, it's just connection pooling with # guarantees against connections idle in # transaction). with base.getTableConn() as conn: subjects = set(r[0] for r in conn.query("SELECT uat_concept" " FROM rr.subject_uat" " WHERE ivoid=%(ivoid)s", locals())) # This is the mapping itself: we do # roots-subjects to avoid adding # root terms that are already in # the record itself. UAT_TOPLEVELS is the result # of the root finding discussed above. for term in subjects: root = UAT_TOPLEVELS[term] newRoots |= (root-subjects) # And finally fiddle in any new root terms found # into the datacite tree if newRoots: subjects = dataciteTree.xpath( "//d:subjects", namespaces={"d": DATACITE_NS})[0] for root in newRoots: newSubject = etree.SubElement(subjects, f"{{{DATACITE_NS}}}subject") newSubject.text = root
Apart from the technicalities I'd again say that's pretty satisfying code.
And these two pieces of code are really all I had to do to map between the vocabularies of different granularities – which I claim will probably be the norm as metadata flows between disciplines.
It's great to see the pieces of a fairly comples puzzle fall into place like that.